The devolution of data
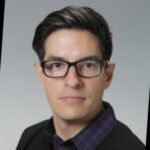
Ian Cowley
Head of Data
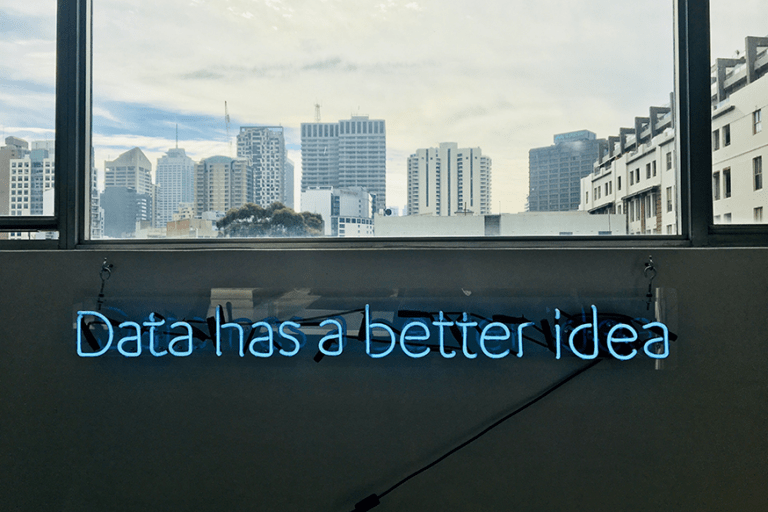
With just about every analytics blog saying data is the new oil, it may come as a surprise to learn that few data projects actually succeed:
- In a Gartner survey in 2016, they estimated 85% of big data projects had failed.
- A four-year study of major analytics initiatives in large companies reported in the Harvard Business Review stated that less than half of the 36 companies studied reported measurable results. Only a third had met their objectives of widespread adoption.
Download our whitepaper to learn how you can enable better data driven decisions in your organisation.
This whitepaper will help you navigate the common pitfalls for data programmes and the structural and cultural changes you can apply to avoid big data problems.
Andrew White, a key Gartner analyst, predicts that it won’t get better any time soon:
“ Through 2022, only 20% of analytic insights will deliver business outcomes”
Bike shedding
What exactly goes wrong then? In my experience people build what they know and even when they haven’t successfully solved their data problem (and it appears few have), they progress to build a monolithic data platform and organisation structure, often without working closely and iteratively with internal partners.
This approach doesn’t encourage flexibility or localised capability. As the organisation isn’t used to dealing with big data problems and the solution has not been born out of organic growth, the data department and surrounding platforms have many of the same problems as legacy MIS (Management Information System) approach. Having spoken to many businesses, the problems are thematic.
1.Poor data literacy
Teams are often unable to understand the data model. Even when there is data cataloguing and strong governance over the available data, without the communication channels, data stewards and engagement with the source teams, the data can feel opaque and meaningless.
It could be that your data team has been diligent in documenting data pipelines, put strict controls around versioning and has good engineering around access control. However, when you contact the data team and ask them what {extraInfoField1} actually does and “it holds a 25 character string” isn’t enough, then you’re in for a three day wait for a service desk ticket to be resolved before you can move on.
What’s more, without someone data centric close to the team, it’s not common for someone to put their hand up and suggest integrating intelligence from other areas, as without the broader knowledge, it’s unlikely to occur to them.
2. Lack of trust in data
A central data team will naturally not be aware of the impact of changes being performed by the various development teams throughout the business and coping with change can be very challenging. Take the following all too common scenario:
The development team, who are actually integrated with a legacy data system, which is unable to support parallel schemas, creates a breaking schema change. The downstream systems aren’t in a place right now to absorb the change and therefore the change needs to be quarantined or delayed.
This is a common scenario, born out of a lack of context, communication and shared responsibility and authority.
3. Difficulty promoting analytics
While analytics and machine learning departments are becoming adept at modelling with big data structures and tooling, it’s rare for them to have a strong engineering background. Therefore, it can be difficult, if not impossible, to productionise their models, often leading to work being left on the shop floor and never seeing the light of day.
What do we actually want from our data and analytics?
I think it’s fair to say that we want to encourage teams to incorporate data centric thinking into their problem solving.
We know there’s a ton of valuable data available throughout the organisation, however operationalising it can be tricky. We want teams to be confident that when they agree to contribute data, it won’t have a drain on their already stretched resources and consequently become a blocker for change and innovation.
We also want to encourage teams to utilise data from other departments, in both their decisions, product and feature justifications and design, as well as to incorporate data streams into their features.
Don’t trust it, won’t use it!
Teams need answers fast. For many of the businesses we have spoken to, the COVID-19 crisis has pointed a sharp lens on the need for fast analytics cycle times. If securing an insight is going to take 3 months, then it could be out of date before you’ve operationalised it.
If the data team has become a bottleneck for progress, people will inevitably start working around the platform. How often have you seen teams create “strategic workarounds”, aka it’s in excel and one person from our department knows how it works, let’s hope they never leave.
Devolution of data
In order to create frictionless access to data and not just connections and catalogues, but a deeper understanding of the value and meaning behind that data, then you need to empower teams. How? By giving them both the authority to change their data and analytics feeds and the responsibility of maintaining them.
“Organizations which design systems are constrained to produce designs which are copies of the communication structures of these organizations”.
– Conways Law
When I first started in development some 21 years ago, in order to get software out the door, you had to get past the QA and Operations trolls, who frequently rejected your work and threw it back over the wall to you.
After a period, we realised this ping pong software process wasn’t working, which gave rise to agile teams and feature squads. Today, it is not unusual to have a team which consists of developers, business analysts, QA and DevOps all working together to get revenue generating features into production quickly.
Data is going through a similar growth spurt, especially with the adoption of DataOps. Creating a data centre of excellence or data practice, which concentrates on moderating, monitoring, supporting and educating, rather than implementing analytics and feeds pushes the responsibility and authority back onto the individual teams.
New roles in the data department
The roles in the data department are growing. This means there is a good chance that different members of the data practice are required at different times, whether it’s DataOps, Data Stewards, Data Engineers or even analytical skills, like Machine Leaning or Data Science. Federating them into the delivery team creates a higher degree of cohesion.
It is important that the data practice participates in sprint ceremonies and design discussions, in the same way as QA or DevOps typically do. By closely integrating the data capability, you are staying core to the agile principle of working together daily throughout the project, welcoming change and delivering frequently.
As they’re federated, members of the data practice still have one hand in the central discipline, meaning they can easily keep up-to-date with central developments and can participate in platform feature election and development.
Changes to infrastructure
It’s important to remember that there will inevitably be infrastructure changes which are required as you go. Processes and capabilities will need to be elevated to reduce the number of repetitive tasks being performed, but the same could be said of any discipline within the software capabilities of your business.
A secondary benefit of this approach (and I’ve seen it work well!) is that the teams incorporate insight and analytics into the way they work, as well as considering the impact of their changes to the data terrain. The difference is felt in sprint planning and design sessions, where changes and features are elected and designed based on data and facts rather than hunches and experience.
Summary
In agile software we want to welcome change and encourage experimentation, but we can’t do that if we have arbitrary bottlenecks. Flow, the state of being in a rhythm of perpetual production only works when your process doesn’t have interrupting hurdles breaking rhythm.
If the aim of your organisation is to become a truly data centric and insight lead business, then building not only the tooling but the right organisational structure to support frictionless access and integration of data and analytic pipelines is critical.
In my time in software and data, I have never seen big up front design work. Iterative design, with fast feedback cycles and the freedom to be creative and innovate, will empower your teams and data departments.
Instead of trying to boil the ocean, start small, iterate often and learn quickly.
Social Share
Don't miss the latest from Ensono
Keep up with Ensono
Innovation never stops, and we support you at every stage. From infrastructure-as-a-service advances to upcoming webinars, explore our news here.
Blog Post | December 18, 2024 | Best practices
Navigating the Complexities of IdAM in Financial Services: A Guide to Choosing the Right Platform
Blog Post | December 17, 2024 | Industry trends
The European Accessibility Act (EAA): Are You Ready for the June 2025 Deadline?
Blog Post | December 5, 2024 | Best practices